Unlocking the Power of Machine Learning Data Labeling
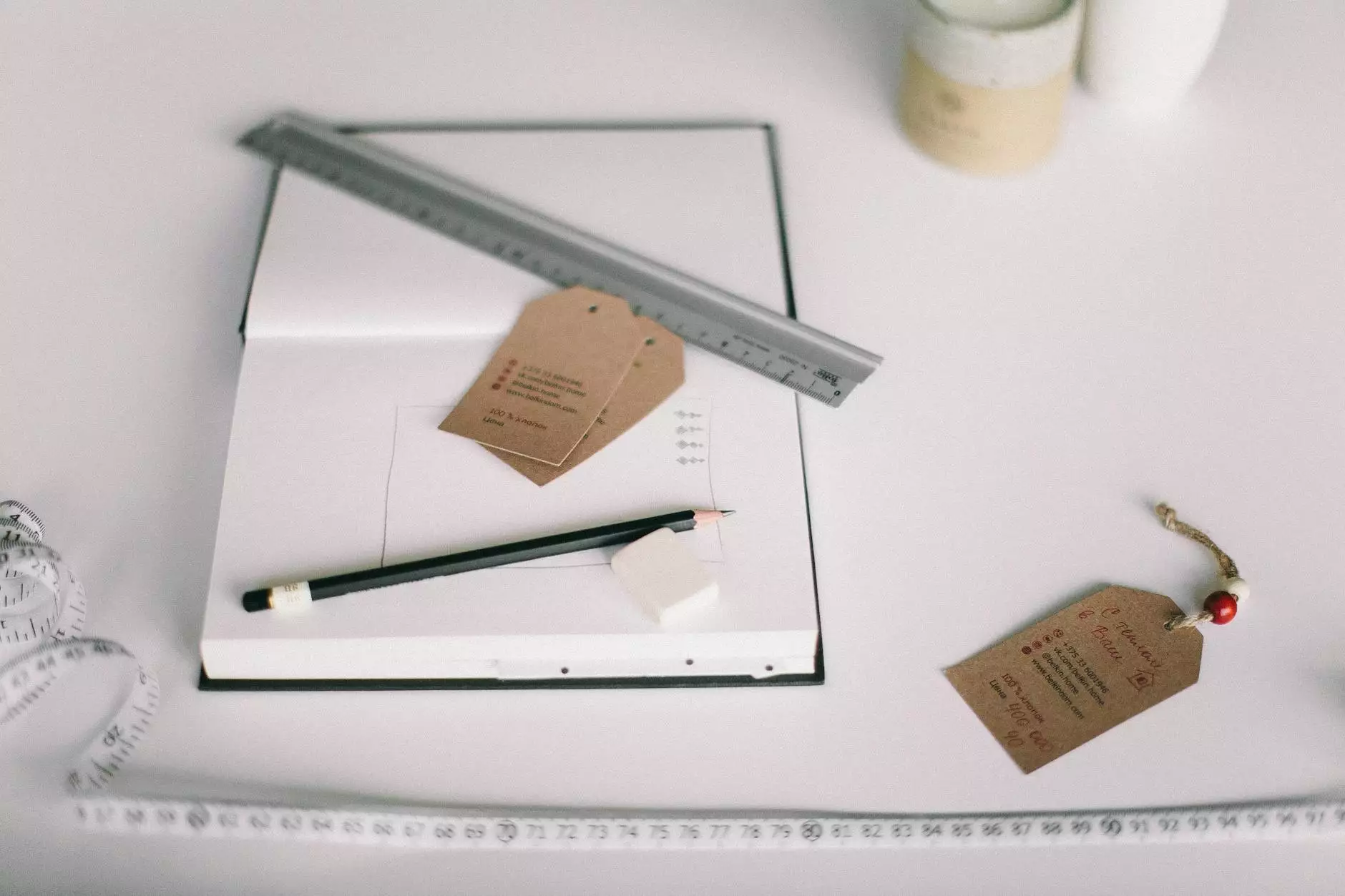
In the rapidly evolving world of artificial intelligence (AI), the importance of machine learning data labeling cannot be overstated. As businesses increasingly rely on AI technologies to automate processes, enhance decision-making, and improve customer experiences, the demand for robust data annotation tools has surged. In this article, we will delve into the intricacies of machine learning data labeling, discuss its critical role in AI development, and highlight how Keylabs.ai offers a comprehensive data annotation platform to meet these demands.
Understanding Machine Learning Data Labeling
At its core, machine learning data labeling is the process of identifying and tagging data to make it comprehensible for AI systems. Data can comprise various formats, including text, images, audio, and video. The labeling process involves assigning meaningful annotations to these datasets, which allows machine learning algorithms to learn from the data and identify patterns.
The Significance of Data Labeling in Machine Learning
Machine learning models rely on high-quality, labeled data to train effectively. Without proper labeling, an AI system will struggle to achieve its intended outcomes. Here are some key benefits:
- Enhanced Accuracy: Labeled data ensures that models can learn with precision, reducing errors in predictions and classifications.
- Faster Training: Accurate labels streamline the training process, allowing algorithms to converge more quickly.
- Improved Performance: High-quality data labeling contributes directly to the performance of machine learning models, enabling better decision-making.
The Machine Learning Data Labeling Process
The data labeling process is multifaceted and can differ depending on the type of data and the application. Here's an overview of the major steps involved:
1. Data Collection
The first step in the data labeling process is collecting relevant data. This data might come from various sources, such as:
- Internal databases
- Public datasets
- Web scraping
- User-generated content
2. Data Preprocessing
Before the actual labeling can occur, it is crucial to preprocess the data. This step may involve:
- Cleaning the data to remove duplicates or irrelevant information
- Normalizing data formats
- Segmenting large datasets into manageable chunks
3. Labeling
During the labeling phase, human annotators or automated tools apply tags and annotations to the data. This can include:
- Bounding box creation for object detection tasks
- Sentiment tagging in textual data
- Image segmentation for image recognition tasks
4. Quality Control
Quality control is a critical component, ensuring accuracy in the annotations. This can involve:
- Reviewing submitted labels by additional annotators
- Utilizing validation datasets to measure label accuracy
- Implementing feedback loops for continuous improvement
5. Model Training
Once the data is accurately labeled, machine learning engineers can begin training their models with the annotated datasets. This process involves feeding labeled data into algorithms to teach them to recognize patterns and make predictions.
Challenges in Machine Learning Data Labeling
Despite its importance, machine learning data labeling is not without its challenges:
1. High Costs
Labeling large datasets can be expensive, especially when hiring skilled annotators. This expense can be prohibitive for small businesses or startups.
2. Time-Intensive
The data labeling process can be slow, particularly for complex data types like video or audio, where each frame or sound byte requires individual attention.
3. Subjectivity
Human labelers may have differing interpretations of data, leading to inconsistencies. Establishing clear guidelines and rigorous quality control is essential to mitigate this issue.
Keylabs.ai: Your Solution for Data Annotation
To address the challenges associated with data labeling, Keylabs.ai offers a cutting-edge data annotation platform that streamlines the labeling process. Here’s how Keylabs.ai stands out:
1. User-Friendly Interface
Keylabs.ai’s platform features an intuitive interface that allows users to easily manage and annotate data. It supports multiple data types, making it versatile for various projects.
2. Advanced Automation
Incorporating AI-driven automation tools, Keylabs.ai can significantly reduce the time spent on data labeling. Automated processes handle repetitive tasks, allowing human annotators to focus on more complex assignments.
3. Quality Assurance Features
The platform includes robust quality assurance tools that enable real-time monitoring of annotations. This ensures that the data produced meets the highest standards of accuracy and reliability.
4. Scalability
Keylabs.ai is designed to scale with your needs. Whether you are a small startup or an established enterprise, the platform can handle any size of dataset while maintaining efficiency.
The Future of Machine Learning Data Labeling
As technologies evolve, the landscape of machine learning data labeling will continue to shift. Here are some trends to watch:
1. Increased Use of AI for Labeling
AI and machine learning will play a more substantial role in automating data labeling, reducing labor costs and increasing speed.
2. Enhanced Collaboration Tools
Future platforms will likely offer enhanced collaboration features, allowing teams to work together more effectively on data annotation projects.
3. Customizable Solutions
Businesses will demand more tailored data annotation tools that cater specifically to their unique needs, leading to greater innovation in the industry.
Conclusion
In conclusion, machine learning data labeling is a critical component of successful AI implementations. As the demand for accurate and reliable labeled data continues to grow, investing in a robust data annotation platform like Keylabs.ai is essential for businesses looking to leverage the power of machine learning. By streamlining the labeling process, ensuring quality, and embracing innovative technologies, Keylabs.ai empowers organizations to thrive in the competitive landscape of AI.